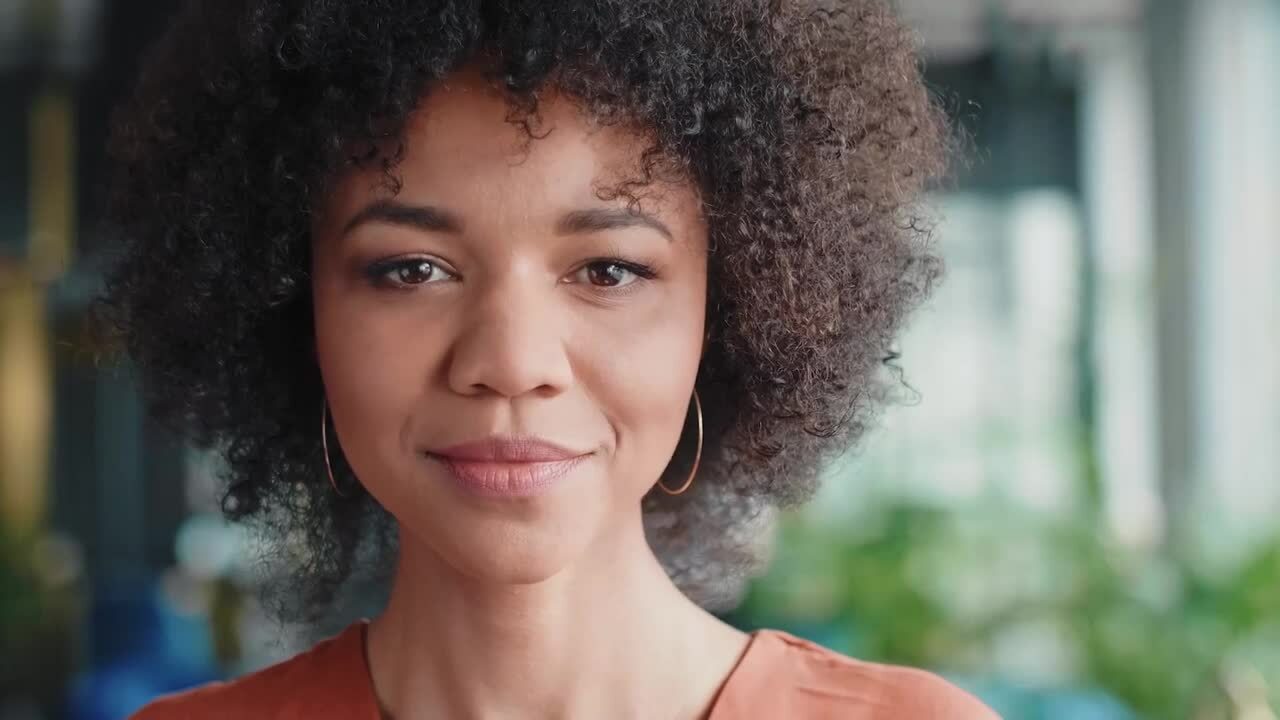
Start understanding
in full color
Leader in 2024 Gartner® Magic Quadrant™ for Voice of the Customer
Human Experience (HX):
A new era for experience & research technology
Trusted by over 2,500 customers, in over 100 countries
Market research
Get to the top with the world’s number 1 market research technology platform. From sample and panel management, comprehensive surveying, advanced analytics, reporting, to data visualization and more. We’re here to help you deliver compelling research, to any requirement.
Experience
Forsta HX is the ultimate Human Experience platform, to help you enhance customer, employee, patient, and local experience. Improve customer retention, drive revenue growth, and enhance operational efficiencies. Gather, analyze, visualize and act on insights, everywhere.
RETAIL HX
Retail is a human business. Power it with with HX.
Pull customer, employee and brand data together. So you get the full picture on cause, effect, and what happens next. Retail HX from Forsta helps reveal the people behind the data points, so you can sell smarter and perform better.
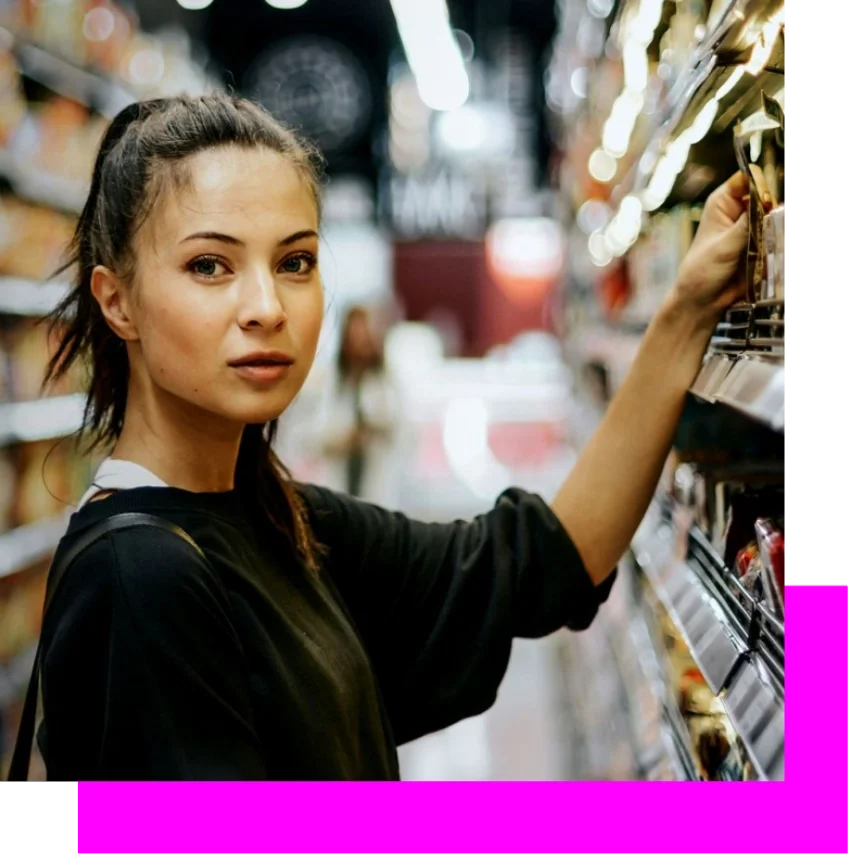
The Forsta HX platform
The most powerful, flexible, connected, and reliable experience & research tech platform. Forsta transcends methodological and data silos. All human experience (HX) is here.
If it’s insightful, it’s measurable. Use customizable surveys to seek insight from any audience – from small teams to global communities. Take the data you need from any touchpoint or channel.
Bring all your data onto a single platform. So you can see the stories behind the statistics. Use advanced analytics tools to search, sort and filter in whatever way gets you to the answers you need.
Put your insights onscreen. Effortlessly. Forsta lets you easily craft all the presentations, reports, infographics and graphs you need. So you can deliver your data as a complete, compelling story.
When the insights are in, make them count. Get the right information to the right people so they can take the right action. Use automation tools to make it instant and effortless.
OUR CUSTOMERS
Trusted by over 2,500 customers,
in over 100 countries.
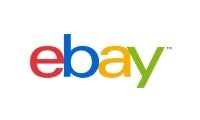
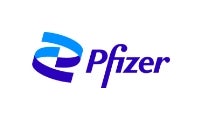

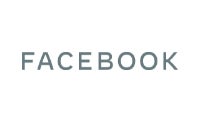
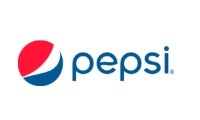


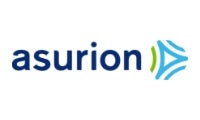
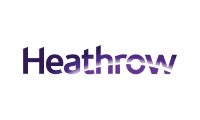
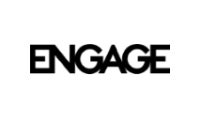
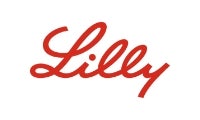
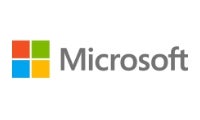
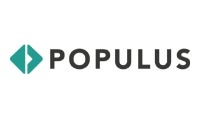
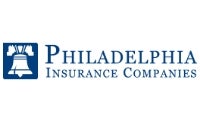
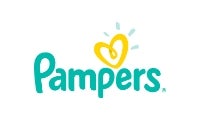
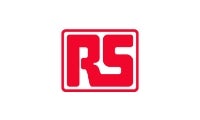
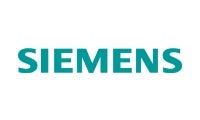
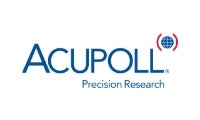

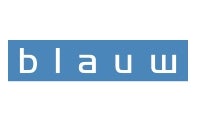
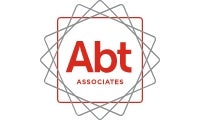
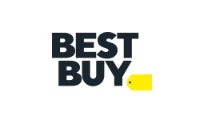


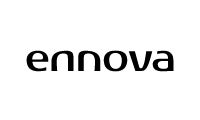
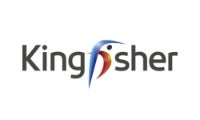
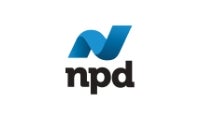
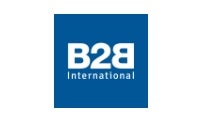
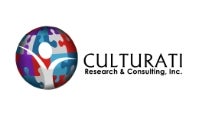
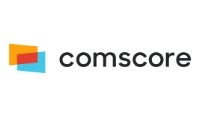
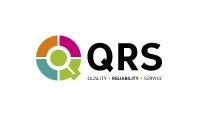
Putting Heathrow on a flight path to discovery
Putting Heathrow on a flight path to discovery Tools used Deck creation Data repository Cross tabulation Infographic dashboards PowerPoint batch reporting Heathrow is Europe’s largest airport. And it collects a lot of information. It interviews 500,000 of the nearly 80 million passengers* who come through its gates every year. Analysing that much data might seem like an impossible […]

Best Buy business success despite the global pandemic
Best Buy business success despite the global pandemic Covid changed so many things in our world beginning in March 2020. Among them, it changed when we could leave our homes, where we went when we did, how we purchased what we needed to live, and how all this was delivered to us. In this webinar, we […]
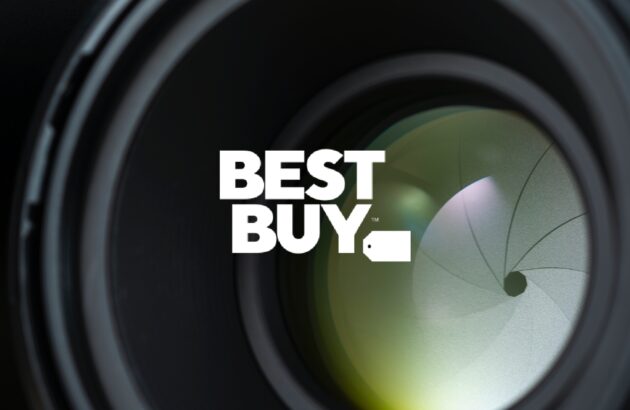